Chatbots and technical writing – Communication is key
It almost always helps when people talk to each other. From a service provider’s perspective, chatbots can be a good way of freeing up staff capacity, particularly for basic questions which take up a lot of time. They are now commonly accepted by customers or people making enquiries and are a useful means of finding out information quickly. In this article we want to highlight how this “chatbot” virtual colleague receives its information and what technical writing has to do with it.
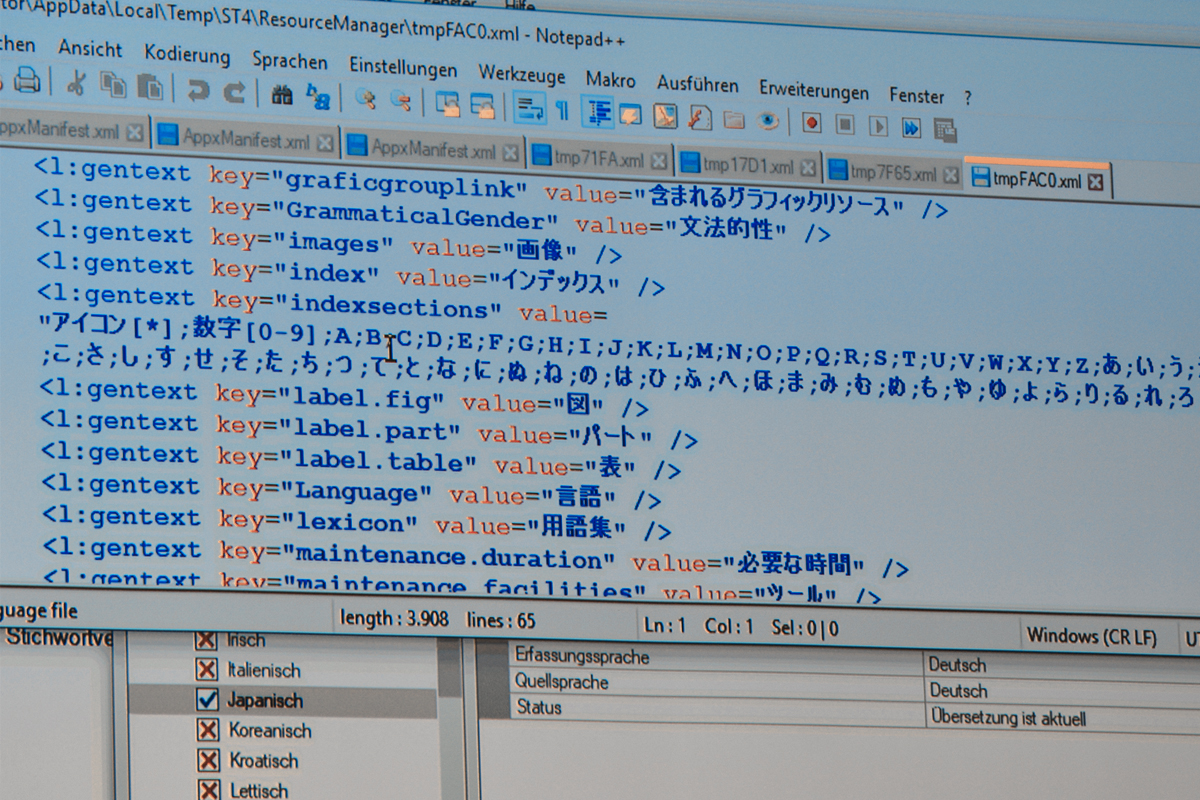
Chatbots – what really happens?
In the best-case scenario, talking to a chatbot works in exactly the same way as talking to a human. You say something, for example, you ask a question, and then the chatbot responds to what you have said, ideally with the correct answer or a follow-up question relating to the solution.
The following steps need to be completed in order for that to happen:
- You ask a question.
- The chatbot takes what you have said as an input.
- The chatbot then identifies a relevant answer.
- The chatbot gives an output and waits for a new input.
Of course, this is only a very rough outline of the process. More in-depth questions such as the following may also arise:
- Are the inputs and outputs written or spoken?
- How exactly are the inputs further analysed in the chatbot?
For outsiders, language analysis is an impenetrable “black box”, which you just have to trust is working properly. For us too this shouldn’t be the main focus: with regards to these purely technical aspects, automated language processing and artificial intelligence have made huge strides (see Siri, Alexa and co.) to enable satisfactory solutions to be found.
There is however one very relevant question that arises regarding answers that are suitable both for the situation and the target group from the asker’s perspective: How does the chatbot identify a relevant answer? Where does it source its information from?
How does the chatbot get its content?
In the example above, the person making the enquiry needs information regarding replacing a valve and is therefore in a maintenance scenario. The valve and the powder coating system are directly affected by this, whereby the valve is part of the system. There are different shapes or variants of both the valve and the system, so the valve needed depends on the type of system.
The chatbot can acquire some information from the input given by the person making the enquiry. If the information provided is insufficient then the chatbot will ask for a clarification, such as “Which valve do you mean?” or “Which system model do you have?”. The context is also often revealed by specific key words, for example, “replace” is a typical keyword to hint at a maintenance scenario.
Other information may already be saved in the chatbot system, such as the following:
- Available system models
- Which (maintainable) parts can be found in these system models
- Whether the pinch valve (type B with the nominal width DN 65) is one of these parts
- Whether it is installed on the suction unit
- The technical data of the valve
- Size (nominal width) of the valve (particularly important here)
If the person making the enquiry responds with anything other than “No” when asked “Do you require help with changing a valve?”, then the chatbot would also have to provide the corresponding operating instructions for this specific maintenance task for the exact model of the system. It is by this stage at the latest that content professionals will notice the correlation between this data and the data which is maintained for technical documentation: a chatbot can only give precise answers if the necessary data is in a modular and classified form.
Classified data for qualified answers
This relates to the scenario we are dealing with here; a fitter, who asks for a particular valve during a specific maintenance activity, requires different answers to an engineer who wants general information about this component during product development. Metadata, which classifies the relevant information module and provides information about its useful usage scenarios (e.g. installation, servicing and maintenance, product information), then determines the situation-specific selection.
For enquiries concerning the selection of components (in our example about the valve, but also about the corresponding system), questions like the following are often asked: To which subcategory of a named category does a product belong (e.g.: Which system version from our product range? Which valve of all the valves installed in the system?)? Taxonomies can help clarify an imprecise input, as they show part-whole relationships or quantity-partial quantity relationships and therefore enable even more detailed responses and accurate content output.
However, it may also be the case that the answers turn out different depending on who asked the question, if for instance administrators should receive different and more specific information to standard users. Such user rights can likewise be defined using metadata.
While certain subtasks of the chatbot conversation can be completed by machines, e.g. the analysis of spoken inputs, the technical writing team is still responsible for the content. They gather, structure and classify the content in such a way that the answers are useful and are as user and situation-specific as the chatbot promises.
For creating and managing such information units, a modern XML-based content management system like SCHEMA ST4 is the method of choice. It already offers all of the possibilities and functionalities which have been mentioned above: modular creation of information units, which can then be used and combined as desired; categorisation of content using metadata; creation and integration of taxonomies to depict relationships. Furthermore, integrated translation management means that even multilingual chatbots are within reach.
The known authoring principle of content structuring, which up until now was “only” used for technical documentation, can therefore also be used to bring additional benefits in other applications.
Other articles from Quanos
This might also interest you
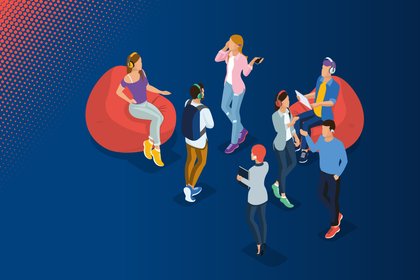
„Doku-Lounge“: Auf dem roten Sofa mit Kerstin Berke und Philipp Eng
Moderatorin Kerstin Berke und Marketingspezialist Philipp Eng sind das Duo vor und hinter dem Mikro der „Doku-Lounge“…