GPT vs. NMT: A Glimpse Into the Future of Translation
Translators have been predicting for some time that their profession will soon become redundant due to AI applications such as ChatGPT. This is surprising since the translation industry in particular has been working with artificial intelligence and machine translation for quite some time. The established NMT tools (neural machine translation systems such as DeepL) are transformer models, similar to the currently much discussed generative AI applications, and have been used on a daily basis in agencies for more than a decade.
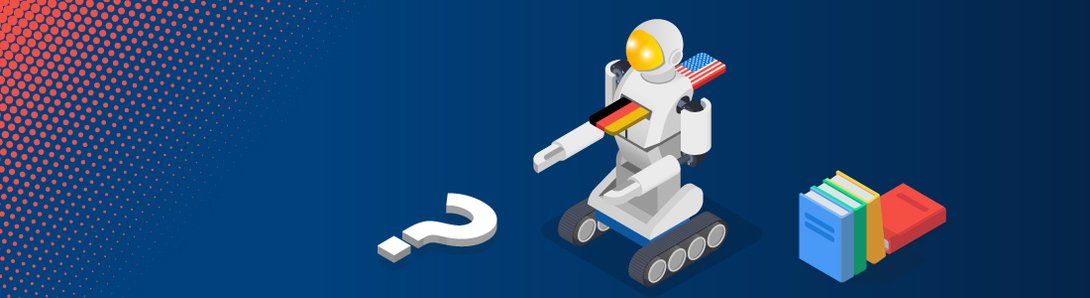
The way translators work, as with almost all knowledge-based professions, is undoubtedly changing. It will shift more towards “post-production”, referred to in the past as “post-editing”. This, too, is nothing new for professional translators, who have been used to receiving suggestions from translation memories, terminology databases, or machine translation engines for decades and using these inputs for their work. With GPT, a new “input provider” has potentially been added here, but it is not yet entirely clear what it can do better than the traditional NMT engines.
NMT or GPT?
So what is the difference between GPT and neural machine translation (NMT), the traditional AI systems that have been used in translation agencies for several years?
Simply put, generative AI systems such as ChatGPT are all-purpose tools. They are trained with a large amount of freely accessible data that is input once. The input for ChatGPT and other generative systems is a prompt which then leads the system to generate a text. What has influenced this output or where the information came from cannot be traced, making it difficult to find a balance between creativity and predictability—which is a problem for many applications in technical writing. This is especially true for ChatGPT, which is explicitly trained to re-enact a “human-like” conversation; in other words, it deliberately does NOT provide consistent output, but varies it. Although this variance can be overridden in a professional application of ChatGPT, these applications are still relatively new and untested, which makes their integration into authoring and translation processes more difficult.
As the name suggests, neural machine translation systems differ in that are specialized in translating texts. They are trained with a bilingual corpus, whereby continuous training is possible and thus adjustments can be made to the system. The input for an NMT system is an entire source text, thus making it much more extensive than, for example, ChatGPT, which is limited to about 4,000 “tokens” as input. The output of NMT is relatively predictable since no new text is created, but “only” existing text is reproduced in another language. Working with NMT is a proven and reliable process, and the systems fit into everyday work in a variety of situations.
What is possible today?
As both systems generate their results based on statistical probabilities, neither truly “understands” what they producing. The results of both AI applications are nevertheless very usable due to the enormous amount of data in the background. In many cases the texts produced can barely be distinguished from texts written by humans,
however errors do occur in both systems (actually even more so in GPT than in NMT) and are difficult to detect. This is because the systems take into account the context of a source text to only a limited extent. This problem is actually even greater with GPT systems as they are always prone to “hallucinations” and completely invent facts.
Incorporating terminology databases is also a problem that can only be partially solved in AI systems. This is due to the fact that GPT engines, in contrast to NMT engines, cannot be “retrained”, but the output can only be adjusted by adding very specific instructions in the prompt. It is therefore difficult for companies to produce a consistent tone of voice and to maintain the company's corporate wording.
To combat this, AI-generated output must always be checked by translation experts or reviewers. They must ensure that the terminology is consistent and that all facts have been translated. With GPT systems, they also have to check that no additional or false information has been added or omitted.
NMT and GPT in use
NMT systems are now routinely used in translation work. They are well integrated into business processes and these systems are precisely tailored to the needs of translation in technical writing departments. Although there is still a need for optimization with NMT (e.g., terminology and tone of voice), there are ingrained processes in place.
For generative models, these processes are still lacking. The results of ChatGPT and other similar systems are easy to read, but at second glance there is much to be desired in terms of quality. Integration into translation tools has also been rudimentary at best. ChatGPT is therefore far better suited to tasks outside of the classic translation process, e.g., to reformulate a text or for the generation of training material for NMT.
But no matter whether NMT or GPT, without human expertise, the results of artificial intelligence cannot be used with confidence. There will ultimately still be a need for translators who operate as a “human expert in the loop” to monitor, repair, and continuously improve the output of the various systems.